Nikolaus Kriegeskorte
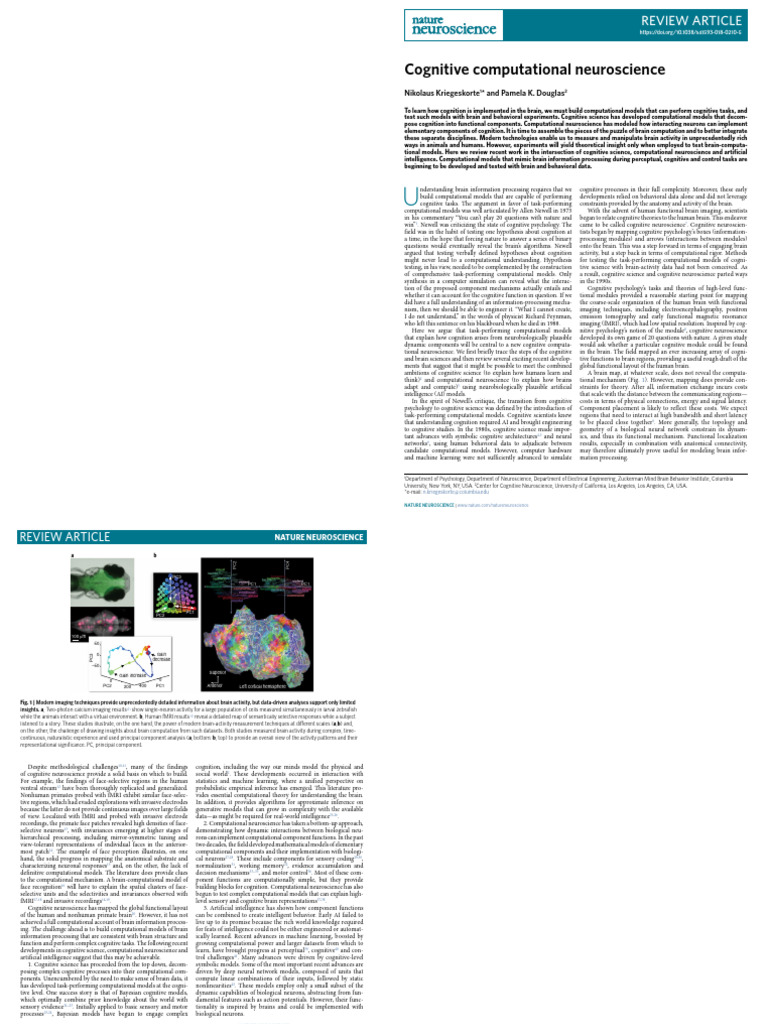
Nikolaus Kriegeskorte, a renowned computational neuroscientist and professor, has made significant contributions to the fields of neuroscience and artificial intelligence. His research focuses on understanding how the human brain processes information and how these insights can be applied to machine learning and cognitive science. With a unique interdisciplinary approach, Kriegeskorte has pioneered innovative methods and theories that bridge the gap between neural activity and computational models, shedding light on the intricate workings of the mind.
Exploring the Brain’s Representational Geometry

Kriegeskorte’s early work centered on the representational geometry of the brain, an innovative concept that involves understanding how different cognitive processes and sensory experiences are represented and organized within neural networks. Through the use of multivariate pattern analysis (MVPA) and representational similarity analysis (RSA), he has been able to visualize and quantify the relationships between various cognitive states and their corresponding neural representations.
One of his key contributions in this area is the development of a theoretical framework known as the hierarchical organization of representational spaces (HORS). HORS proposes that the brain's representational geometry is structured in a hierarchical manner, with different levels of abstraction and specificity. This framework has been instrumental in understanding how the brain processes and integrates information from various sensory modalities and cognitive domains.
Functional MRI and MVPA
Kriegeskorte’s laboratory has extensively utilized functional magnetic resonance imaging (fMRI) in conjunction with MVPA to study the brain’s representational geometry. By analyzing patterns of neural activity across different brain regions, his team has been able to decode and compare the representations of various cognitive processes, shedding light on the brain’s complex information processing mechanisms.
Study Focus | Key Findings |
---|---|
Visual Object Recognition | Demonstrated that object representations in the brain follow a hierarchical structure, with increasing levels of abstraction in higher visual areas. |
Categorization and Decision-Making | Identified distinct neural representations for different decision-making strategies, suggesting a flexible and adaptive categorization system in the brain. |
Cross-Modal Representations | Found evidence of shared representational spaces between visual and auditory modalities, indicating that the brain integrates information across senses. |

Machine Learning and Neural Networks

Kriegeskorte’s research has also made significant inroads into the field of machine learning and artificial neural networks. By applying his understanding of the brain’s representational geometry to machine learning algorithms, he has been able to develop more biologically plausible and efficient models.
Deep Neural Networks
In collaboration with other researchers, Kriegeskorte has trained deep neural networks to perform various cognitive tasks, such as object recognition and natural language processing. These networks, inspired by the brain’s hierarchical organization, have demonstrated remarkable performance, rivaling or even surpassing traditional machine learning models.
One notable achievement is the development of a deep neural network that can identify and classify visual objects with a high degree of accuracy. This network, modeled after the brain's visual processing hierarchy, has provided valuable insights into the computational principles underlying visual perception.
Biologically Inspired Algorithms
Kriegeskorte has also explored the development of novel machine learning algorithms that are directly inspired by the brain’s computational strategies. These algorithms, while being efficient and effective, also adhere to the biological constraints and principles observed in neural systems.
Algorithm | Inspiration | Application |
---|---|---|
Neuromodulated Adaptive Resonance Theory (NART) | Inspired by the brain's neuromodulatory systems | Fast and accurate pattern recognition, especially in noisy environments |
Self-Organizing Neural Networks | Modeled after the brain's self-organizing capabilities | Unsupervised learning and clustering of complex data |
Spiking Neural Networks | Mimicking the brain's temporal dynamics | Efficient processing of temporal sequences and event prediction |
Cognitive Neuroscience and Clinical Applications
Beyond its implications for machine learning, Kriegeskorte’s research has also had a significant impact on cognitive neuroscience and clinical practice. By understanding the brain’s representational geometry, researchers and clinicians can gain insights into various cognitive and neurological disorders, leading to improved diagnosis and treatment strategies.
Neuropsychiatric Disorders
Kriegeskorte’s team has applied their methods to study the neural representations associated with various neuropsychiatric disorders, such as schizophrenia and autism spectrum disorders. By comparing the representational geometry of affected individuals with that of healthy controls, they have been able to identify distinctive patterns that may serve as biomarkers for these conditions.
For instance, in a study on schizophrenia, they found that the representational geometry of the default mode network (a brain network associated with self-referential processing) was altered in individuals with the disorder. This finding not only provides a potential neural marker for schizophrenia but also offers insights into the underlying cognitive impairments associated with the disease.
Cognitive Rehabilitation
Kriegeskorte’s work has also informed cognitive rehabilitation strategies. By understanding how the brain represents and processes information, clinicians can design more effective interventions to improve cognitive functioning in individuals with brain injuries or neurodegenerative diseases.
For example, his team has developed a cognitive training program based on the brain's representational geometry. This program, which targets specific neural representations, has shown promising results in improving cognitive performance in individuals with mild cognitive impairment.
Future Directions and Impact
Kriegeskorte’s research continues to push the boundaries of our understanding of the brain and its computational capabilities. As he and his colleagues further explore the brain’s representational geometry and its implications for machine learning and cognitive science, we can expect significant advancements in various fields, including artificial intelligence, cognitive neuroscience, and clinical practice.
Moreover, the interdisciplinary nature of Kriegeskorte's work fosters collaboration between neuroscientists, computer scientists, and clinicians, fostering a more holistic and integrated approach to understanding the mind and its disorders. This collaborative spirit is essential for tackling complex neurological and psychiatric conditions and has the potential to revolutionize the way we approach brain-related research and healthcare.
What is the main focus of Nikolaus Kriegeskorte’s research?
+Kriegeskorte’s research primarily focuses on understanding the brain’s representational geometry and its implications for machine learning and cognitive science. He aims to bridge the gap between neural activity and computational models, providing insights into how the brain processes information.
How does Kriegeskorte study the brain’s representational geometry?
+Kriegeskorte utilizes a range of techniques, including functional MRI (fMRI) and multivariate pattern analysis (MVPA), to study the brain’s representational geometry. These methods allow him to visualize and quantify the relationships between cognitive processes and their corresponding neural representations.
What are some of the key applications of Kriegeskorte’s work in machine learning?
+Kriegeskorte’s work has led to the development of more biologically plausible and efficient machine learning models. His biologically inspired algorithms offer a promising direction for creating robust and adaptable systems, with potential applications in robotics, autonomous systems, and various other fields.
How does Kriegeskorte’s research impact cognitive neuroscience and clinical practice?
+By understanding the brain’s representational geometry, Kriegeskorte’s research provides insights into various cognitive and neurological disorders. His work has identified distinctive neural patterns associated with conditions like schizophrenia and autism spectrum disorders, offering potential biomarkers and informing cognitive rehabilitation strategies.