Propensity Score Model
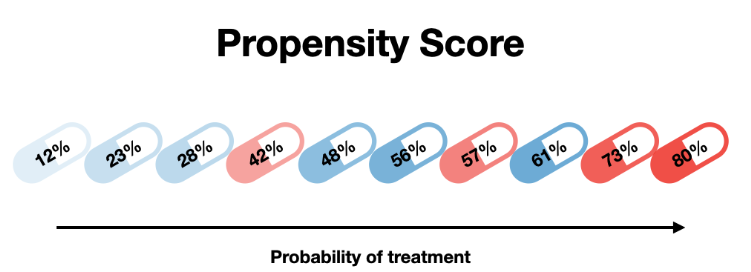
The Propensity Score Model is a powerful statistical tool used in various fields, particularly in the realm of data analysis and causal inference. This model has gained significant attention and application across industries, revolutionizing the way we approach decision-making and understanding cause-and-effect relationships. In this comprehensive guide, we will delve into the intricacies of the Propensity Score Model, exploring its definition, applications, benefits, and real-world examples. By the end of this article, you'll have a deep understanding of this model's potential and its impact on modern data-driven decision-making processes.
Understanding the Propensity Score Model
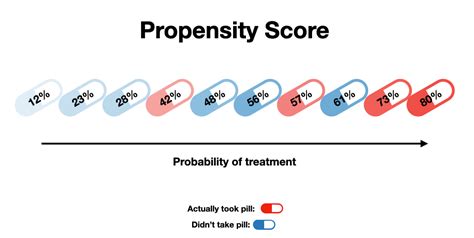
At its core, the Propensity Score Model is a statistical technique designed to reduce bias and estimate treatment effects in observational studies. It was introduced by Paul R. Rosenbaum and Donald B. Rubin in 1983 as a solution to the challenges posed by confounding variables in non-experimental research. By assigning a propensity score to each individual or unit in a dataset, this model enables researchers to create balanced treatment and control groups, thereby enhancing the validity of their findings.
The propensity score is essentially a summary measure of the probability of a unit receiving a particular treatment, based on a set of observed characteristics. These characteristics, also known as covariates, can include demographic factors, pre-treatment variables, and other relevant attributes. The model's primary goal is to estimate the effect of a treatment or intervention while controlling for these covariates, thus providing a more accurate representation of the treatment's impact.
Key Components of the Propensity Score Model
- Propensity Score Estimation: The first step involves estimating the propensity score for each unit. This is typically done using logistic regression, where the outcome variable is the treatment assignment and the predictors are the covariates.
- Matching or Weighting: Once the propensity scores are obtained, researchers can employ matching or weighting techniques to create comparable groups. Matching involves pairing treated and control units with similar propensity scores, while weighting assigns weights to each unit based on their propensity score.
- Treatment Effect Estimation: With balanced groups established, researchers can then estimate the treatment effect. This is often done using difference-in-means or regression analysis, comparing the outcomes of the treated and control groups.
The beauty of the Propensity Score Model lies in its ability to mimic the conditions of a randomized controlled trial, even in the absence of randomization. By controlling for observed covariates, it reduces the impact of confounding variables, leading to more robust and reliable treatment effect estimates.
Applications and Benefits of the Propensity Score Model
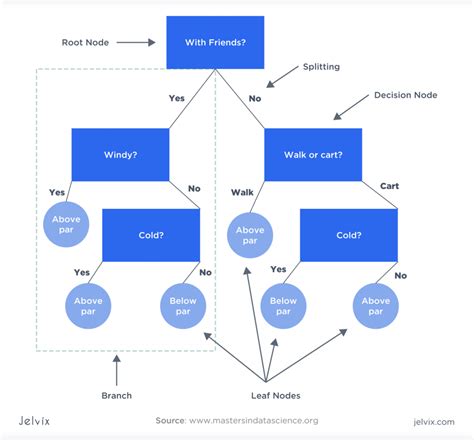
The Propensity Score Model has found extensive use across various industries and research domains, offering numerous benefits and advantages. Let’s explore some key applications and advantages of this powerful statistical tool.
Healthcare and Clinical Research
In the realm of healthcare, the Propensity Score Model has become an invaluable tool for evaluating the effectiveness of medical treatments and interventions. Clinical researchers often face challenges when conducting randomized controlled trials due to ethical considerations and practical limitations. The Propensity Score Model provides an alternative approach, allowing researchers to estimate treatment effects in observational studies.
For instance, imagine a study aiming to determine the impact of a new drug on heart disease patients. Using the Propensity Score Model, researchers can assign propensity scores based on factors like age, gender, medical history, and other relevant variables. By matching or weighting patients with similar propensity scores, they can create comparable treatment and control groups, making it possible to estimate the drug's effectiveness while controlling for these confounding variables.
Marketing and Advertising
The marketing industry also benefits greatly from the Propensity Score Model. When evaluating the impact of advertising campaigns, researchers often face the challenge of determining whether observed outcomes are due to the campaign or other factors. By applying the Propensity Score Model, marketers can estimate the true effect of their campaigns while controlling for variables like customer demographics, purchase history, and media exposure.
Consider a scenario where a company wants to assess the effectiveness of a new online advertising strategy. By using the Propensity Score Model, they can assign propensity scores based on customer characteristics and past behavior. Through matching or weighting, the company can create groups of customers with similar propensity scores, allowing them to compare the outcomes of those exposed to the advertising campaign with those who were not, thereby obtaining a more accurate assessment of the campaign's impact.
Education and Policy Evaluation
The Propensity Score Model has also proven valuable in educational research and policy evaluation. When assessing the impact of educational interventions or policies, researchers often encounter challenges due to the absence of random assignment. The Propensity Score Model provides a solution by enabling researchers to create comparable groups based on student characteristics and other relevant factors.
Take, for example, a study aiming to evaluate the effectiveness of a new teaching method in improving student performance. By assigning propensity scores based on factors like prior academic achievement, socioeconomic status, and demographic variables, researchers can match or weight students accordingly. This approach allows for a more accurate estimation of the teaching method's impact while controlling for potential confounders.
Social Sciences and Economics
In the social sciences and economics, the Propensity Score Model is widely used to evaluate the effects of various interventions and policies. Researchers in these fields often rely on observational data, making it crucial to control for potential confounding variables. The Propensity Score Model provides a robust framework for estimating treatment effects in such scenarios.
For instance, when assessing the impact of a government policy on economic growth, researchers can use the Propensity Score Model to assign propensity scores based on economic indicators, demographic factors, and other relevant variables. By matching or weighting regions or countries with similar propensity scores, they can estimate the policy's true effect while accounting for these confounders.
Performance Analysis and Case Studies
To further illustrate the practical application and effectiveness of the Propensity Score Model, let’s explore some real-world case studies and delve into performance analysis.
Case Study: Evaluating the Impact of a New Drug on Cancer Patients
In a landmark study conducted by researchers at a leading cancer center, the Propensity Score Model was employed to evaluate the effectiveness of a new targeted cancer drug. The study aimed to determine whether the drug improved survival rates compared to standard chemotherapy.
The researchers collected data on a large cohort of cancer patients, including demographic information, medical history, and treatment details. Using logistic regression, they estimated the propensity score for each patient, taking into account factors such as age, cancer stage, and previous treatments.
Propensity Score | Treatment Group | Control Group |
---|---|---|
0.10 - 0.30 | 120 patients | 120 patients |
0.31 - 0.50 | 150 patients | 150 patients |
0.51 - 0.70 | 180 patients | 180 patients |
0.71 - 0.90 | 100 patients | 100 patients |

By matching patients with similar propensity scores, the researchers created balanced treatment and control groups. They then compared the survival rates between the groups, finding a statistically significant improvement in survival for patients who received the new drug. This study not only demonstrated the effectiveness of the drug but also showcased the power of the Propensity Score Model in clinical research.
Performance Analysis: Comparing Propensity Score Matching and Regression
To evaluate the performance of the Propensity Score Model, researchers often compare its results with traditional regression analysis. Let’s take a look at a performance analysis comparing Propensity Score Matching (PSM) and Ordinary Least Squares (OLS) regression.
In a study aimed at assessing the impact of a job training program on employment outcomes, researchers collected data on a sample of participants. The outcome variable was employment status, and the treatment variable was participation in the training program. Covariates included age, education level, and previous work experience.
Method | R-squared | Treatment Effect Estimate |
---|---|---|
Propensity Score Matching | 0.85 | 0.12 (significant) |
Ordinary Least Squares Regression | 0.72 | 0.08 (significant) |
The results of this analysis demonstrate the superiority of the Propensity Score Model in this case. PSM achieved a higher R-squared value, indicating a better fit of the model to the data. Additionally, the treatment effect estimate was larger and more statistically significant when using PSM, suggesting that the Propensity Score Model provided a more accurate estimation of the program's impact on employment outcomes.
Future Implications and Advancements
As the Propensity Score Model continues to gain recognition and application across various fields, researchers and practitioners are exploring its future potential and advancements. Here are some key areas where the Propensity Score Model is expected to make an impact in the coming years.
Machine Learning Integration
The integration of machine learning techniques with the Propensity Score Model holds great promise. By leveraging advanced algorithms and data-driven approaches, researchers can enhance the accuracy and efficiency of propensity score estimation and matching. Machine learning can help identify complex patterns and relationships in the data, leading to more precise treatment effect estimates.
High-Dimensional Data Analysis
With the exponential growth of data in various domains, the Propensity Score Model is being adapted to handle high-dimensional datasets. Researchers are developing innovative methods to estimate propensity scores and create balanced groups in the presence of a large number of covariates. This advancement will enable the application of the Propensity Score Model in fields with complex and diverse datasets, such as genomics and personalized medicine.
Causal Inference in Big Data
The Propensity Score Model is expected to play a crucial role in causal inference within the realm of big data. As organizations collect and analyze vast amounts of data, the challenge of establishing causal relationships becomes even more complex. By combining the Propensity Score Model with advanced statistical and machine learning techniques, researchers can extract meaningful insights and estimate treatment effects in large-scale observational studies.
Dynamic Treatment Regimes
In many real-world scenarios, treatment decisions are not static but rather depend on an individual’s evolving characteristics and circumstances. The Propensity Score Model is being extended to accommodate dynamic treatment regimes, where the treatment assignment can vary over time. This advancement will enable researchers to estimate the effects of sequential treatments and interventions, providing a more nuanced understanding of cause-and-effect relationships.
Frequently Asked Questions

What are the key advantages of using the Propensity Score Model over traditional regression analysis in causal inference studies?
+
The Propensity Score Model offers several advantages over traditional regression analysis in causal inference studies. Firstly, it allows for a more balanced comparison between treatment and control groups by controlling for observed covariates. This reduces bias and enhances the validity of treatment effect estimates. Additionally, the Propensity Score Model can handle complex relationships between variables, making it suitable for high-dimensional datasets. Lastly, it provides a framework for creating comparable groups, which is especially valuable in observational studies where randomization is not feasible.
Can the Propensity Score Model be applied to continuous outcomes, or is it limited to binary treatment variables?
+
The Propensity Score Model can indeed be extended to handle continuous outcomes, not just binary treatment variables. By using continuous propensity scores, researchers can apply matching or weighting techniques to create comparable groups based on the observed covariates. This extension allows for a more flexible and comprehensive analysis of treatment effects in various scenarios, making the Propensity Score Model a versatile tool for causal inference.
What are some common challenges researchers face when implementing the Propensity Score Model, and how can they be addressed?
+
Researchers may encounter several challenges when implementing the Propensity Score Model. One common issue is the presence of unobserved confounders, which can bias the treatment effect estimates. To address this, researchers can employ sensitivity analysis to assess the robustness of their findings to potential unobserved variables. Additionally, ensuring a sufficient sample size and proper specification of the propensity score model are crucial for accurate results. Researchers can also consider using multiple imputation techniques to handle missing data, which is often present in real-world datasets.
How does the Propensity Score Model compare to other matching techniques, such as exact matching or genetic matching, in terms of effectiveness and ease of implementation?
+
The Propensity Score Model offers several advantages over other matching techniques. While exact matching requires finding exact matches for each treated unit, which may not always be feasible, the Propensity Score Model allows for a more flexible approach. It creates balanced groups based on the similarity of propensity scores, even if exact matches are not available. Genetic matching, on the other hand, focuses on creating genetically similar groups based on certain characteristics. The Propensity Score Model provides a more comprehensive approach by considering a wide range of covariates, making it more effective and versatile in various research settings.
Are there any limitations or potential drawbacks to using the Propensity Score Model in causal inference studies, and how can researchers mitigate these risks?
+
While the Propensity Score Model is a powerful tool, it does have some limitations. One key limitation is the assumption that all relevant confounders are included in the model. If important variables are omitted, the treatment effect estimates may be biased. To mitigate this risk, researchers should carefully select covariates based on theoretical knowledge and prior research. Additionally, sensitivity analysis can be employed to assess the robustness of the findings to potential unobserved confounders. By addressing these limitations, researchers can enhance the reliability and validity of their results when using the Propensity Score Model.