Rich Zemel

Richard "Rich" Zemel is a renowned Canadian computer scientist and Professor Emeritus at the University of Toronto, recognized for his groundbreaking contributions to the fields of machine learning, artificial intelligence, and statistics. His research has significantly shaped the development of modern AI and deep learning algorithms, influencing a wide range of applications from natural language processing to computer vision.
With a career spanning over three decades, Zemel's expertise lies in the theoretical foundations and practical applications of machine learning, particularly in the context of learning with structured data and probabilistic graphical models. His work has been instrumental in advancing the understanding and development of machine learning techniques, making him a highly respected figure in the international AI research community.
In this comprehensive article, we delve into the extraordinary career of Rich Zemel, exploring his influential research, significant contributions to the field, and his role in shaping the future of AI. We will examine his seminal works, notable achievements, and the profound impact he has had on the evolution of machine learning and its real-world applications.
The Early Years: Education and Early Career

Rich Zemel’s journey into the world of computer science and artificial intelligence began with a solid foundation in mathematics and theoretical research. Born and raised in Canada, he developed a keen interest in mathematics and its applications early on. This passion led him to pursue a Bachelor of Science degree in Mathematics and Computer Science at the University of Toronto, where he graduated with distinction.
Following his undergraduate studies, Zemel continued his academic journey by enrolling in the prestigious University of California, Berkeley for his graduate studies. Here, he embarked on a Ph.D. program in Computer Science, specializing in Machine Learning and Artificial Intelligence. Under the mentorship of renowned AI researchers, Zemel delved deep into the theoretical aspects of machine learning, developing innovative algorithms and techniques that laid the groundwork for his future research contributions.
During his Ph.D. studies, Zemel's research focused on the development of efficient algorithms for learning with structured data, particularly in the context of probabilistic graphical models. His work during this period laid the foundation for many of the advancements he would later make in the field of machine learning. Upon completing his Ph.D., Zemel joined the faculty at the University of Toronto, where he has spent the majority of his career advancing the frontiers of AI research.
Research Contributions: Shaping the Future of Machine Learning

Rich Zemel’s research career has been characterized by a relentless pursuit of innovative solutions to some of the most challenging problems in machine learning and artificial intelligence. His contributions have not only advanced the theoretical foundations of these fields but have also paved the way for practical applications across a wide range of industries.
Learning with Structured Data
One of Zemel’s key areas of expertise is learning with structured data, a domain that has become increasingly important in the era of big data. Structured data, such as graphs, trees, and networks, is ubiquitous in many real-world applications, from social networks and transportation systems to biological networks. Zemel’s research has focused on developing efficient and effective algorithms for learning from these complex data structures, enabling machines to better understand and analyze the intricate relationships within structured data.
A notable contribution in this area is his work on graphical models, which provide a probabilistic framework for representing and reasoning about complex dependencies in structured data. Zemel's algorithms for learning in graphical models have been instrumental in improving the accuracy and efficiency of machine learning tasks, particularly in fields like natural language processing and computer vision, where structured data is prevalent.
Probabilistic Graphical Models and Their Applications
Probabilistic graphical models have been a cornerstone of Zemel’s research, and his contributions to this field have been extensive. These models provide a powerful tool for representing and reasoning about uncertainty in complex systems, making them invaluable in a wide range of AI applications.
Zemel's work has focused on developing efficient inference algorithms for graphical models, which are essential for making predictions and decisions in real-world scenarios. His algorithms have been applied to various domains, including medical diagnosis, image analysis, and natural language understanding. For example, in medical diagnosis, graphical models can represent the complex relationships between symptoms, diseases, and patient data, enabling more accurate and personalized healthcare decisions.
Deep Learning and Representation Learning
As deep learning emerged as a dominant force in AI, Zemel played a pivotal role in advancing this field. His research has focused on understanding the theoretical foundations of deep learning and developing new algorithms for representation learning, a key component of deep neural networks.
Zemel's work in representation learning has aimed to address the challenge of learning meaningful representations of data that can be used for various downstream tasks. His contributions have led to the development of more robust and efficient deep learning models, which have found applications in areas such as image and speech recognition, natural language processing, and robotics.
Transfer Learning and Domain Adaptation
Another area where Zemel’s research has made significant contributions is transfer learning and domain adaptation. Transfer learning involves applying knowledge learned from one domain to another, while domain adaptation focuses on adapting models to new domains with different distributions. These techniques are crucial for leveraging existing knowledge and data to solve new problems efficiently.
Zemel's research has explored how to effectively transfer knowledge across different domains, particularly in the context of structured data. His algorithms for domain adaptation have been applied to various real-world problems, such as adapting models trained on one language to work effectively on another, or adapting models trained on synthetic data to real-world scenarios.
Awards and Recognition: A Testimony to Excellence
Rich Zemel’s outstanding contributions to the field of machine learning and artificial intelligence have been recognized through numerous awards and honors. His work has not only advanced the theoretical understanding of these fields but has also led to practical advancements with real-world impact.
ACM Fellow
In 2018, Zemel was inducted as a Fellow of the Association for Computing Machinery (ACM), one of the highest honors in the field of computing. This recognition is a testament to his significant contributions to the theory and practice of machine learning, particularly in the areas of probabilistic graphical models and learning with structured data.
Best Paper Awards
Zemel’s research has been consistently recognized at top-tier conferences and journals in the field of machine learning. He has received multiple best paper awards, including the prestigious Neural Information Processing Systems (NeurIPS) Best Paper Award in 2019 for his work on domain adaptation in deep learning.
Invited Talks and Keynotes
Zemel’s expertise and contributions have made him a sought-after speaker at international conferences and workshops. He has delivered invited talks and keynotes at prestigious venues, sharing his insights and research with the global AI community. His talks have covered a wide range of topics, from the theoretical foundations of machine learning to the practical applications of AI in various industries.
Impact on Industry and Real-World Applications
Zemel’s research has not only influenced the academic community but has also had a significant impact on industry and real-world applications. His algorithms and techniques have been adopted by leading technology companies and research institutions, contributing to advancements in areas such as natural language processing, computer vision, and robotics.
For example, Zemel's work on learning with structured data has been instrumental in developing more accurate and efficient models for social network analysis, recommendation systems, and personalized advertising. His contributions to probabilistic graphical models have improved the performance of medical diagnosis systems and image analysis tools, leading to better healthcare outcomes and more accurate image recognition.
Mentorship and Impact on the Next Generation of Researchers
In addition to his research accomplishments, Rich Zemel has made significant contributions to the development and mentoring of the next generation of AI researchers. His dedication to education and mentorship has helped shape the careers of countless students and researchers, ensuring the continued growth and innovation in the field of machine learning.
Teaching Excellence
Zemel’s commitment to education is evident in his teaching career at the University of Toronto. He has taught a wide range of courses in machine learning, artificial intelligence, and statistics, inspiring and guiding students towards their own research paths. His teaching methods have been praised for their clarity and ability to engage students, making complex concepts accessible and exciting.
Supervising Graduate Students
As a supervisor, Zemel has guided numerous graduate students through their research journeys, providing valuable insights and mentorship. His students have gone on to make their own contributions to the field, continuing the cycle of knowledge and innovation. Zemel’s approach to supervision focuses on fostering independence and creativity, encouraging students to explore new ideas and approaches while providing guidance and support.
Collaborations and Research Partnerships
Zemel’s collaborative spirit has led to numerous research partnerships and collaborations, both within the University of Toronto and with other institutions worldwide. These collaborations have resulted in groundbreaking research and the development of innovative solutions to complex problems. By working together, Zemel and his colleagues have pushed the boundaries of what is possible in machine learning and artificial intelligence.
The Future of AI: Rich Zemel’s Vision and Perspective
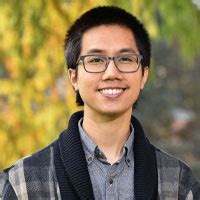
As one of the leading experts in machine learning and artificial intelligence, Rich Zemel offers a unique perspective on the future of these fields. With his deep understanding of the theoretical foundations and practical applications of AI, he provides valuable insights into the challenges and opportunities that lie ahead.
Continued Advancements in Machine Learning
Zemel believes that the future of machine learning lies in continued advancements in theoretical understanding and practical applications. He sees a need for further development of efficient algorithms and techniques that can handle the increasing complexity of real-world data and tasks. This includes improving the scalability and robustness of machine learning models, particularly in the context of learning with structured data and large-scale datasets.
Ethical Considerations and AI Responsibility
As AI becomes increasingly integrated into our lives, Zemel emphasizes the importance of ethical considerations and responsible AI development. He advocates for the need to address biases and fairness in machine learning algorithms, ensuring that AI systems are transparent, accountable, and beneficial to society. This includes addressing issues such as algorithmic bias, privacy concerns, and the responsible use of AI in sensitive domains like healthcare and finance.
The Role of AI in Addressing Global Challenges
Zemel is optimistic about the potential of AI to address some of the world’s most pressing challenges. He believes that AI can play a crucial role in fields such as healthcare, education, and environmental sustainability. For example, AI-powered systems can assist in diagnosing and treating diseases, improving educational outcomes, and optimizing resource management for a more sustainable future.
The Impact of AI on Society and the Economy
Zemel acknowledges the transformative impact of AI on society and the economy. He emphasizes the need for a balanced approach to AI adoption, ensuring that the benefits are distributed equitably and that potential risks are mitigated. This includes addressing issues such as job displacement, digital inequality, and the responsible development and regulation of AI technologies.
Conclusion: A Legacy of Innovation and Impact
Rich Zemel’s career has been a testament to the power of innovative thinking and dedicated research. His contributions to the fields of machine learning and artificial intelligence have been instrumental in shaping the future of these technologies, influencing the way we interact with and understand the world around us.
Through his research, Zemel has advanced the theoretical foundations of machine learning, developed practical algorithms for real-world applications, and guided the next generation of AI researchers. His work continues to inspire and inform, pushing the boundaries of what is possible and driving the field forward.
As we look to the future, Rich Zemel's vision and perspective provide a roadmap for the continued development and responsible adoption of AI technologies. His legacy of innovation and impact will undoubtedly continue to shape the world of artificial intelligence for years to come.
What is Rich Zemel’s primary area of research expertise?
+Rich Zemel’s primary area of research expertise is machine learning, particularly learning with structured data and probabilistic graphical models.
How has Zemel’s research impacted the field of AI?
+Zemel’s research has advanced the theoretical foundations of AI and developed practical algorithms for learning with structured data, probabilistic graphical models, and deep learning. His work has led to more accurate and efficient machine learning models, impacting various real-world applications.
What are some of Zemel’s notable awards and recognitions?
+Zemel has received numerous awards, including being inducted as a Fellow of the Association for Computing Machinery (ACM) and receiving best paper awards at top-tier conferences like NeurIPS.
How does Zemel approach mentorship and the development of the next generation of AI researchers?
+Zemel believes in fostering independence and creativity in his students, providing guidance and support while encouraging them to explore new ideas and approaches. He has supervised numerous graduate students and has a reputation for excellent teaching and mentorship.